In our previous study (Betancourt et al. 2021), we published a benchmark dataset on ozone metrics extracted from the TOAR database along with a machine learning task. We want to tackle the challenge with machine learning to predict the ozone metrics based upon geospatial features. As a baseline, we predicted, for example, the average ozone with different methods, among them a random forest and a shallow neural network.
Although many studies, like ours, reach their goal by just making the predictions – we asked more questions. How exactly do the machine learning algorithms predict average ozone? We analyzed our machine learning models’ functioning to understand their predictions and found out even more than we expected.
By focusing on inaccurate predictions and explaining why these predictions fail, we (i) identified underrepresented samples, (ii) flagged unexpected inaccurate predictions, and (iii) pointed out training samples irrelevant for predictions. We suggested locations for building new measurement stations based on the underrepresented samples. We also showed which training samples do not substantially contribute to the model performance. We can even drop these samples without performance loss! Our study demonstrates the application of explainable machine learning beyond simply explaining the trained model.
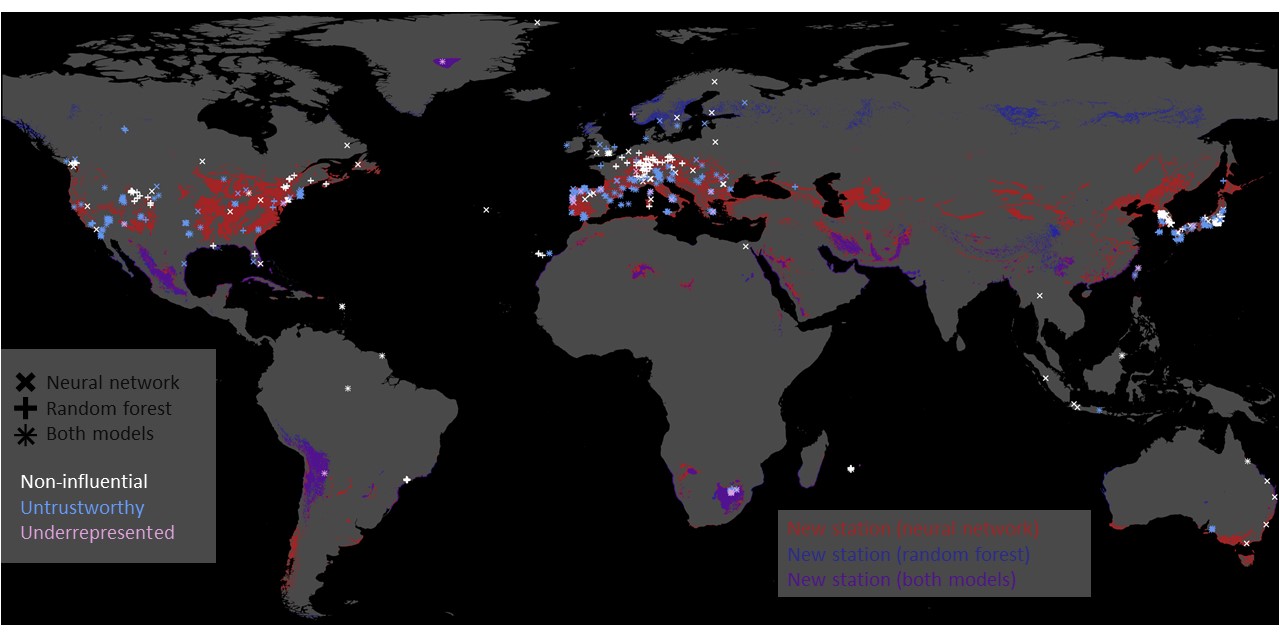
Stadtler et al., Explainable Machine Learning Reveals Capabilities, Redundancy, and Limitations of a Geospatial Air Quality Benchmark Dataset, Machine Learning and Knowledge Extraction. 2022; 4(1):150-171, https://doi.org/10.3390/make4010008