Lukas H. Leufen, Felix Kleinert (both FZ Jülich and University of Bonn) and Martin G. Schultz (FZ Jülich) have published their latest research results of the study “Exploring decomposition of temporal patterns to facilitate learning of neural networks for ground-level daily maximum 8-hour average ozone prediction” in the Journal Environmental Data Science. The study shows how the accuracy of deep neural networks for forecasting ground-level ozone can be improved by splitting long-term and short-term weather patterns. The article is available at https://www.doi.org/10.1017/eds.2022.9 .
Author: Lukas Leufen
GeoDataServices
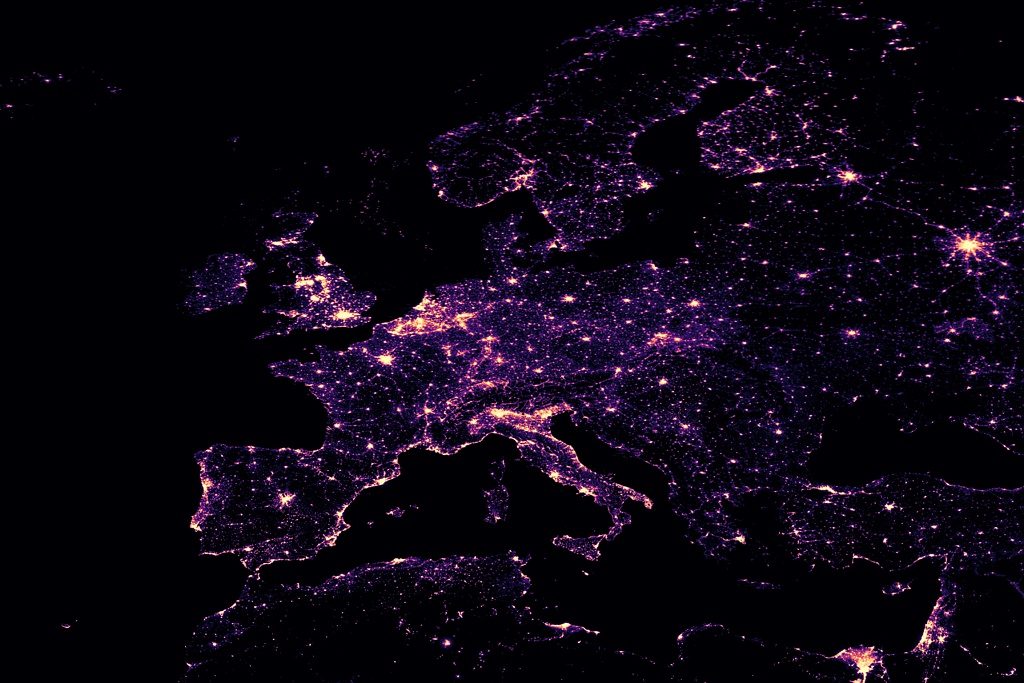
With GeoDataServices (Schultz, M.G. et.al., 2018), we enable an automated and flexible characterisation of an arbitrary point location using high resolution data. These services are accessible through a standardised REST API and can therefore easily be used by both human and machine. In the current version, GeoDataServices includes geographical information on topography and dominant land surface covers, anthropogenic data about urbanisation (human settlements, built-up areas, nighttime light brightness, population density and streets) and agriculture yields (rice and wheat), climatological and environmental data as NOx emissions and climatic zones. Combining this data, GeoDataServices can characterise any point location and therefore enables users to compare locations in a personalised -use case driven- way. For this personalisation, each query needs to be specified with a radius around the point location, in which a given statistical aggregation function is applied. Beside this personalised information preparation, the GeoDataServices are also included in the TOAR database metadata creation. GeoDataServices is currently under development and not yet accessible for the public. However, interested people are encouraged to contact us for more insight into GeoDataServices.
Reference: Schultz, Martin G., et al. “A web service architecture for objective station classification purposes.” 2018 IEEE 14th International Conference on e-Science (e-Science). IEEE, 2018.